正確さと高速化に成功したYOLO V3
こんにちは。
AI coordinator管理人の清水秀樹です。
最近はラズパイにハマってdeeplearningの勉強をサボっておりましたが、YOLO V2をさらに高速化させたYOLO V3がリリースされたようなので、早速試してみました。
しかもより正確になったようです。
開発環境
- imac2012 27-inch
- macOS High Sierra
- Python 3.5.2
- PyTorch
- Anaconda使用
- GPUは使用しない
公式サイトからのダウロード
YOLO V3を使うだけならすぐにできます。
公式サイトを参考に以下の通りにコマンドラインをただ入力するだけ試すことができます。
$ git clone https://github.com/pjreddie/darknet $ cd darknet $ make $ wget https://pjreddie.com/media/files/yolov3.weights $ ./darknet detect cfg/yolov3.cfg yolov3.weights data/dog.jpg
すると
layer filters size input output 0 conv 32 3 x 3 / 1 416 x 416 x 3 -> 416 x 416 x 32 0.299 BFLOPs 1 conv 64 3 x 3 / 2 416 x 416 x 32 -> 208 x 208 x 64 1.595 BFLOPs 2 conv 32 1 x 1 / 1 208 x 208 x 64 -> 208 x 208 x 32 0.177 BFLOPs 3 conv 64 3 x 3 / 1 208 x 208 x 32 -> 208 x 208 x 64 1.595 BFLOPs 4 res 1 208 x 208 x 64 -> 208 x 208 x 64 5 conv 128 3 x 3 / 2 208 x 208 x 64 -> 104 x 104 x 128 1.595 BFLOPs 6 conv 64 1 x 1 / 1 104 x 104 x 128 -> 104 x 104 x 64 0.177 BFLOPs 7 conv 128 3 x 3 / 1 104 x 104 x 64 -> 104 x 104 x 128 1.595 BFLOPs 8 res 5 104 x 104 x 128 -> 104 x 104 x 128 9 conv 64 1 x 1 / 1 104 x 104 x 128 -> 104 x 104 x 64 0.177 BFLOPs 10 conv 128 3 x 3 / 1 104 x 104 x 64 -> 104 x 104 x 128 1.595 BFLOPs 11 res 8 104 x 104 x 128 -> 104 x 104 x 128 12 conv 256 3 x 3 / 2 104 x 104 x 128 -> 52 x 52 x 256 1.595 BFLOPs 13 conv 128 1 x 1 / 1 52 x 52 x 256 -> 52 x 52 x 128 0.177 BFLOPs 14 conv 256 3 x 3 / 1 52 x 52 x 128 -> 52 x 52 x 256 1.595 BFLOPs 15 res 12 52 x 52 x 256 -> 52 x 52 x 256 16 conv 128 1 x 1 / 1 52 x 52 x 256 -> 52 x 52 x 128 0.177 BFLOPs 17 conv 256 3 x 3 / 1 52 x 52 x 128 -> 52 x 52 x 256 1.595 BFLOPs 18 res 15 52 x 52 x 256 -> 52 x 52 x 256 19 conv 128 1 x 1 / 1 52 x 52 x 256 -> 52 x 52 x 128 0.177 BFLOPs 20 conv 256 3 x 3 / 1 52 x 52 x 128 -> 52 x 52 x 256 1.595 BFLOPs 21 res 18 52 x 52 x 256 -> 52 x 52 x 256 22 conv 128 1 x 1 / 1 52 x 52 x 256 -> 52 x 52 x 128 0.177 BFLOPs 23 conv 256 3 x 3 / 1 52 x 52 x 128 -> 52 x 52 x 256 1.595 BFLOPs 24 res 21 52 x 52 x 256 -> 52 x 52 x 256 25 conv 128 1 x 1 / 1 52 x 52 x 256 -> 52 x 52 x 128 0.177 BFLOPs 26 conv 256 3 x 3 / 1 52 x 52 x 128 -> 52 x 52 x 256 1.595 BFLOPs 27 res 24 52 x 52 x 256 -> 52 x 52 x 256 28 conv 128 1 x 1 / 1 52 x 52 x 256 -> 52 x 52 x 128 0.177 BFLOPs 29 conv 256 3 x 3 / 1 52 x 52 x 128 -> 52 x 52 x 256 1.595 BFLOPs 30 res 27 52 x 52 x 256 -> 52 x 52 x 256 31 conv 128 1 x 1 / 1 52 x 52 x 256 -> 52 x 52 x 128 0.177 BFLOPs 32 conv 256 3 x 3 / 1 52 x 52 x 128 -> 52 x 52 x 256 1.595 BFLOPs 33 res 30 52 x 52 x 256 -> 52 x 52 x 256 34 conv 128 1 x 1 / 1 52 x 52 x 256 -> 52 x 52 x 128 0.177 BFLOPs 35 conv 256 3 x 3 / 1 52 x 52 x 128 -> 52 x 52 x 256 1.595 BFLOPs 36 res 33 52 x 52 x 256 -> 52 x 52 x 256 37 conv 512 3 x 3 / 2 52 x 52 x 256 -> 26 x 26 x 512 1.595 BFLOPs 38 conv 256 1 x 1 / 1 26 x 26 x 512 -> 26 x 26 x 256 0.177 BFLOPs 39 conv 512 3 x 3 / 1 26 x 26 x 256 -> 26 x 26 x 512 1.595 BFLOPs 40 res 37 26 x 26 x 512 -> 26 x 26 x 512 41 conv 256 1 x 1 / 1 26 x 26 x 512 -> 26 x 26 x 256 0.177 BFLOPs 42 conv 512 3 x 3 / 1 26 x 26 x 256 -> 26 x 26 x 512 1.595 BFLOPs 43 res 40 26 x 26 x 512 -> 26 x 26 x 512 44 conv 256 1 x 1 / 1 26 x 26 x 512 -> 26 x 26 x 256 0.177 BFLOPs 45 conv 512 3 x 3 / 1 26 x 26 x 256 -> 26 x 26 x 512 1.595 BFLOPs 46 res 43 26 x 26 x 512 -> 26 x 26 x 512 47 conv 256 1 x 1 / 1 26 x 26 x 512 -> 26 x 26 x 256 0.177 BFLOPs 48 conv 512 3 x 3 / 1 26 x 26 x 256 -> 26 x 26 x 512 1.595 BFLOPs 49 res 46 26 x 26 x 512 -> 26 x 26 x 512 50 conv 256 1 x 1 / 1 26 x 26 x 512 -> 26 x 26 x 256 0.177 BFLOPs 51 conv 512 3 x 3 / 1 26 x 26 x 256 -> 26 x 26 x 512 1.595 BFLOPs 52 res 49 26 x 26 x 512 -> 26 x 26 x 512 53 conv 256 1 x 1 / 1 26 x 26 x 512 -> 26 x 26 x 256 0.177 BFLOPs 54 conv 512 3 x 3 / 1 26 x 26 x 256 -> 26 x 26 x 512 1.595 BFLOPs 55 res 52 26 x 26 x 512 -> 26 x 26 x 512 56 conv 256 1 x 1 / 1 26 x 26 x 512 -> 26 x 26 x 256 0.177 BFLOPs 57 conv 512 3 x 3 / 1 26 x 26 x 256 -> 26 x 26 x 512 1.595 BFLOPs 58 res 55 26 x 26 x 512 -> 26 x 26 x 512 59 conv 256 1 x 1 / 1 26 x 26 x 512 -> 26 x 26 x 256 0.177 BFLOPs 60 conv 512 3 x 3 / 1 26 x 26 x 256 -> 26 x 26 x 512 1.595 BFLOPs 61 res 58 26 x 26 x 512 -> 26 x 26 x 512 62 conv 1024 3 x 3 / 2 26 x 26 x 512 -> 13 x 13 x1024 1.595 BFLOPs 63 conv 512 1 x 1 / 1 13 x 13 x1024 -> 13 x 13 x 512 0.177 BFLOPs 64 conv 1024 3 x 3 / 1 13 x 13 x 512 -> 13 x 13 x1024 1.595 BFLOPs 65 res 62 13 x 13 x1024 -> 13 x 13 x1024 66 conv 512 1 x 1 / 1 13 x 13 x1024 -> 13 x 13 x 512 0.177 BFLOPs 67 conv 1024 3 x 3 / 1 13 x 13 x 512 -> 13 x 13 x1024 1.595 BFLOPs 68 res 65 13 x 13 x1024 -> 13 x 13 x1024 69 conv 512 1 x 1 / 1 13 x 13 x1024 -> 13 x 13 x 512 0.177 BFLOPs 70 conv 1024 3 x 3 / 1 13 x 13 x 512 -> 13 x 13 x1024 1.595 BFLOPs 71 res 68 13 x 13 x1024 -> 13 x 13 x1024 72 conv 512 1 x 1 / 1 13 x 13 x1024 -> 13 x 13 x 512 0.177 BFLOPs 73 conv 1024 3 x 3 / 1 13 x 13 x 512 -> 13 x 13 x1024 1.595 BFLOPs 74 res 71 13 x 13 x1024 -> 13 x 13 x1024 75 conv 512 1 x 1 / 1 13 x 13 x1024 -> 13 x 13 x 512 0.177 BFLOPs 76 conv 1024 3 x 3 / 1 13 x 13 x 512 -> 13 x 13 x1024 1.595 BFLOPs 77 conv 512 1 x 1 / 1 13 x 13 x1024 -> 13 x 13 x 512 0.177 BFLOPs 78 conv 1024 3 x 3 / 1 13 x 13 x 512 -> 13 x 13 x1024 1.595 BFLOPs 79 conv 512 1 x 1 / 1 13 x 13 x1024 -> 13 x 13 x 512 0.177 BFLOPs 80 conv 1024 3 x 3 / 1 13 x 13 x 512 -> 13 x 13 x1024 1.595 BFLOPs 81 conv 255 1 x 1 / 1 13 x 13 x1024 -> 13 x 13 x 255 0.088 BFLOPs 82 detection 83 route 79 84 conv 256 1 x 1 / 1 13 x 13 x 512 -> 13 x 13 x 256 0.044 BFLOPs 85 upsample 2x 13 x 13 x 256 -> 26 x 26 x 256 86 route 85 61 87 conv 256 1 x 1 / 1 26 x 26 x 768 -> 26 x 26 x 256 0.266 BFLOPs 88 conv 512 3 x 3 / 1 26 x 26 x 256 -> 26 x 26 x 512 1.595 BFLOPs 89 conv 256 1 x 1 / 1 26 x 26 x 512 -> 26 x 26 x 256 0.177 BFLOPs 90 conv 512 3 x 3 / 1 26 x 26 x 256 -> 26 x 26 x 512 1.595 BFLOPs 91 conv 256 1 x 1 / 1 26 x 26 x 512 -> 26 x 26 x 256 0.177 BFLOPs 92 conv 512 3 x 3 / 1 26 x 26 x 256 -> 26 x 26 x 512 1.595 BFLOPs 93 conv 255 1 x 1 / 1 26 x 26 x 512 -> 26 x 26 x 255 0.177 BFLOPs 94 detection 95 route 91 96 conv 128 1 x 1 / 1 26 x 26 x 256 -> 26 x 26 x 128 0.044 BFLOPs 97 upsample 2x 26 x 26 x 128 -> 52 x 52 x 128 98 route 97 36 99 conv 128 1 x 1 / 1 52 x 52 x 384 -> 52 x 52 x 128 0.266 BFLOPs 100 conv 256 3 x 3 / 1 52 x 52 x 128 -> 52 x 52 x 256 1.595 BFLOPs 101 conv 128 1 x 1 / 1 52 x 52 x 256 -> 52 x 52 x 128 0.177 BFLOPs 102 conv 256 3 x 3 / 1 52 x 52 x 128 -> 52 x 52 x 256 1.595 BFLOPs 103 conv 128 1 x 1 / 1 52 x 52 x 256 -> 52 x 52 x 128 0.177 BFLOPs 104 conv 256 3 x 3 / 1 52 x 52 x 128 -> 52 x 52 x 256 1.595 BFLOPs 105 conv 255 1 x 1 / 1 52 x 52 x 256 -> 52 x 52 x 255 0.353 BFLOPs 106 detection Loading weights from yolov3.weights...Done! data/dog.jpg: Predicted in 9.686850 seconds. truck: 92% bicycle: 99% dog: 99%
結果が表示されます。
物体検出した肝心の画像はdarknetフォルダ内に保存されています。
YOLOといえばこの画像が王道ですね。
公式サイトには次々と連続して画像を読み込ませる方法の紹介もありますし、閾値を変更して物体検出する方法なども紹介されていますので、興味がある方は試してみると良いかと思います。
PyTorchを使ったリアルタイム映像での物体検出
続いてカメラ映像から試してみたいと思います。
今回は最近出てきたPyTorchを使って物体検出を試してみたいと思います。
GitHubにソースが公開されていたので、ありがたく使用させて頂きます。
$ git clone https://github.com/ayooshkathuria/pytorch-yolo-v3.git $ cd pytorch-yolo-v3 $ wget https://pjreddie.com/media/files/yolov3.weights $ python detect.py --images imgs --det det
この段階で以下のようなエラーが出た方はPyTorchがインストールされていないことによるエラーです。
python: can't open file 'detect.py': [Errno 2] No such file or directory
PyTorch公式サイトにインストール方法が紹介されています。
OSやパッケージマネージャやPythonのバージョンとCUDAの有無を選択すると、Run this commandにインストールできるコマンドが表示されます。
筆者の環境ではAnacondaを使用していますので、condaでインストールしました。
$ conda install pytorch torchvision -c pytorch
インストール後に再度実行してみましょう。
$ python detect.py --images imgs --det det
次々と結果が表示されれば成功です。
Loading network..... Network successfully loaded dog.jpg predicted in 0.986 seconds Objects Detected: bicycle truck dog ---------------------------------------------------------- eagle.jpg predicted in 1.028 seconds Objects Detected: bird ---------------------------------------------------------- giraffe.jpg predicted in 1.024 seconds Objects Detected: zebra giraffe giraffe ---------------------------------------------------------- herd_of_horses.jpg predicted in 1.123 seconds Objects Detected: horse horse horse horse ---------------------------------------------------------- img1.jpg predicted in 1.036 seconds Objects Detected: person dog ---------------------------------------------------------- img2.jpg predicted in 1.026 seconds Objects Detected: train ---------------------------------------------------------- img3.jpg predicted in 1.070 seconds Objects Detected: car car car car car car car truck traffic light ---------------------------------------------------------- img4.jpg predicted in 1.023 seconds Objects Detected: chair chair chair clock ---------------------------------------------------------- messi.jpg predicted in 1.054 seconds Objects Detected: person person person frisbee ---------------------------------------------------------- person.jpg predicted in 1.005 seconds Objects Detected: person dog horse ---------------------------------------------------------- scream.jpg predicted in 1.153 seconds Objects Detected: bed ---------------------------------------------------------- SUMMARY ---------------------------------------------------------- Task : Time Taken (in seconds) Reading addresses : 0.001 Loading batch : 1.639 Detection (11 images) : 11.529 Output Processing : 0.000 Drawing Boxes : 0.181 Average time_per_img : 1.214 ----------------------------------------------------------
動画は以下のコマンドで実行しましょう。
$ python video_demo.py --video video.MOV
は、早い!!!!!!
すげー
CPUなのにかなり早い。
ちょっと感動です。
内蔵カメラでも以下のコマンドで確認可能です。
$ python cam_demo.py
は、は、早い!!!!
かなりの高速化を体感できます。
ハードの性能が変わらないのに、これだけ高速化できるのは本当にすごいと思います。
技術の進歩は本当に早いですね。
ぜひ試してみてください。
それではまた。
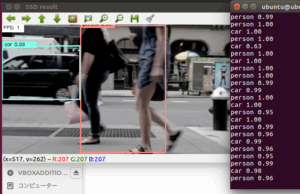
その他の物体検出記事はこちらから
初めまして。
YOLOなど使ったシステム構築を考えています。
ご相談したいこと御座いますので、メールにて相談致したくご連絡頂ければ幸です。
何卒宜しくお願い致します。